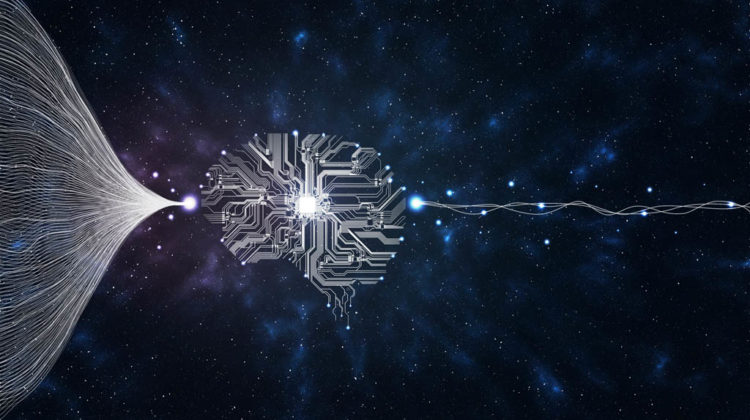
A team of researchers at Pennsylvania State University has developed a method to account for the effect of small defects in ultrathin interfaces known as optical metasurfaces before they’ve occurred, enabling designers to work around the associated performance reductions.
Optical metasurfaces, which are made up of uniform nanoscale structures, change the behaviour of light waves that hit them to produce effects that range from unique reflection and transmission properties to the removal of lens distortion. However, because metasurface features are so tiny, any manufacturing defects can significantly reduce their performance — and both the defects themselves and their effects are difficult to anticipate.
‘With modern nanofabrication technology, superfine features – or small structures inside metasurface components – can be made consistently, but this can affect the processing time,’ said Ronald Jenkins, an electrical engineering doctoral candidate at Penn State. ‘The goal of this research was to identify a method that would allow design of structures that can tolerate those inevitable defects and still perform well.’
The researchers first generate metasurface designs using an optimiser – software that tests a range of different designs and then suggests new variations based on the most successful configurations. These data were used as inputs in a deep-learning model that had been trained to predict how inevitable manufacturing defects would affect their performance using data from highly accurate, electromagnetic modelling software.
The deep learning model applied its trained knowledge of how defects influence performance to determine the robustness of the designs created by the optimiser to those defects. This assessment was then fed back into to the optimiser, which then generated high-performance designs that could withstand the effect of manufacturing defects as described by the deep learning model.
‘It would take an overwhelming computational load to complete both design optimisation and integration of the uncertainty arising from manufacturing in a conventional model,’ Jenkins said. ‘That’s where the deep learning comes in – to tell the optimiser how well its design performed so it can more intelligently select the next iteration.’
This approach also allowed the researchers to facilitate the design of new shapes for the metasurface components. ‘In the past, the unit cells making up metasurfaces have been restricted to simpler shapes, such as squares or circles, to reduce the chance of developing a defect during fabrication that can hinder performance,’ said Sawyer Campbell, an assistant research professor in Penn State’s School of Electrical Engineering and Computer Science. ‘By implementing fabrication errors on the simulation side in advance, we can enable more freeform designs that have been shown in simulations to achieve extremely high performance.’
According to the researchers, high-performance metasurfaces featuring unusual and desirable properties that result from light interactions at the nanoscale could improve a variety of technologies. Metasurfaces can bend light waves, for example, enabling the production of flat lenses that are significantly smaller than conventional optical lenses.
They also pointed out that the deep learning and optimisation strategies they employed could be applied to many other areas. ‘This concept could be adapted to a wide range of engineering problems,’ Campbell said. ‘Deep learning is an emerging field in optics and electromagnetics, and Ron is taking it in a new direction.’
The research has been published in Nanophotonics.